CTE-POWER User's Guide
Table of Contents
- Introduction
- System Overview
- Compiling applications
- Connecting to CTE-POWER
- File Systems
- Data management
- Running Jobs
- Software Environment
- Getting help
- Frequently Asked Questions (FAQ)
- Appendices
Introduction ↩
This user’s guide for the CTE IBM Power9 cluster is intended to provide the minimum amount of information needed by a new user of this system. As such, it assumes that the user is familiar with many of the standard features of supercomputing as the Unix operating system.
Here you can find most of the information you need to use our computing resources and the technical documentation about the machine. Please read carefully this document and if any doubt arises do not hesitate to contact us (Getting help).
System Overview ↩
CTE-POWER is a cluster based on IBM Power9 processors, with a Linux Operating System and an Infiniband interconnection network.
It has the following configuration:
- 2 login node and 52 compute nodes, each of them:
- 2 x IBM Power9 8335-GTH @ 2.4GHz (3.0GHz on turbo, 20 cores and 4 threads/core, total 160 threads per node)
- 512GB of main memory distributed in 16 dimms x 32GB @ 2666MHz
- 2 x SSD 1.9TB as local storage
- 2 x 3.2TB NVME
- 4 x GPU NVIDIA V100 (Volta) with 16GB HBM2.
- Single Port Mellanox EDR
- GPFS via one fiber link 10 GBit
The operating system is Red Hat Enterprise Linux Server 7.5 alternative.
Other relevant information ↩
- CUDA version: 10.1
- CUDA driver: 418.39
- Mellanox IB 4.3–4
- IBM Firmware: 920.10
The interconnection between CPU and GPU is done using NVLink 2.0.
Compiling applications ↩
For compiling applications the system provides GCC version 4.8.5, IBM XL compilers for C/C++ v13.1.6 and for Fortran v15.1.6.
Via modules you can find other compilers such as:
- GCC/7.3.0
- PGI/18.1
Connecting to CTE-POWER ↩
The first thing you should know is your username and password. Once you have a login and its associated password you can get into the cluster through the following login nodes:
- plogin1.bsc.es
- plogin2.bsc.es
This will provide you with a shell in the login node. There you can compile and prepare your applications.
You must use Secure Shell (ssh) tools to login into or transfer files into the cluster. We do not accept incoming connections from protocols like telnet, ftp, rlogin, rcp, or rsh commands. Once you have logged into the cluster you cannot make outgoing connections for security reasons.
Password Management ↩
In order to change the password, you have to login to a different machine (dt01.bsc.es). This connection must be established from your local machine.
% ssh -l username dt01.bsc.es
username@dtransfer1:~> passwd
Changing password for username.
Old Password:
New Password:
Reenter New Password:
Password changed.
Mind that the password change takes about 10 minutes to be effective.
File Systems ↩
IMPORTANT:* It is your responsibility as a user of our facilities to backup all your critical data. We only guarantee a daily backup of user data under /gpfs/home. Any other backup should only be done exceptionally under demand of the interested user.
Each user has several areas of disk space for storing files. These areas may have size or time limits, please read carefully all this section to know about the policy of usage of each of these filesystems. There are 4 different types of storage available inside a node:
- GPFS filesystems: GPFS is a distributed networked filesystem which can be accessed from all the nodes and Data Transfer Machine
- Local hard drive: Every node has an internal hard drive
- Root filesystem: Is the filesystem where the operating system resides
- NVMe: Non-Volatile Memory Express local partition in every node.
GPFS Filesystem ↩
The IBM General Parallel File System (GPFS) is a high-performance shared-disk file system providing fast, reliable data access from all nodes of the cluster to a global filesystem. GPFS allows parallel applications simultaneous access to a set of files (even a single file) from any node that has the GPFS file system mounted while providing a high level of control over all file system operations. In addition, GPFS can read or write large blocks of data in a single I/O operation, thereby minimizing overhead.
An incremental backup will be performed daily only for /gpfs/home.
These are the GPFS filesystems available in the machine from all nodes:
/apps: Over this filesystem will reside the applications and libraries that have already been installed on the machine. Take a look at the directories to know the applications available for general use.
/gpfs/home: This filesystem has the home directories of all the users, and when you log in you start in your home directory by default. Every user will have their own home directory to store own developed sources and their personal data. A default quota will be enforced on all users to limit the amount of data stored there. Also, it is highly discouraged to run jobs from this filesystem. Please run your jobs on your group’s /gpfs/projects or /gpfs/scratch instead.
/gpfs/projects: In addition to the home directory, there is a directory in /gpfs/projects for each group of users. For instance, the group bsc01 will have a /gpfs/projects/bsc01 directory ready to use. This space is intended to store data that needs to be shared between the users of the same group or project. A quota per group will be enforced depending on the space assigned by Access Committee. It is the project’s manager responsibility to determine and coordinate the better use of this space, and how it is distributed or shared between their users.
/gpfs/scratch: Each user will have a directory over /gpfs/scratch. Its intended use is to store temporary files of your jobs during their execution. A quota per group will be enforced depending on the space assigned.
Active Archive - HSM (Tape Layer) ↩
Active Archive (AA) is a mid-long term storage filesystem that provides 15 PB of total space. You can access AA from the Data Transfer Machine (dt01.bsc.es and dt02.bsc.es) under /gpfs/archive/hpc/your_group.
NOTE: There is no backup of this filesystem. The user is responsible for adequately managing the data stored in it.
Hierarchical Storage Management (HSM) is a data storage technique that automatically moves data between high-cost and low-cost storage media. At BSC, the filesystem using HSM is the one mounted at /gpfs/archive/hpc, and the two types of storage are GPFS (high-cost, low latency) and Tapes (low-cost, high latency).
HSM System Overview
Hardware
- IBM TS4500 with 10 Frames
- 6000 Tapes 12TB LTO8
- 64 Drives
- 8 LC9 Power9 Servers
Software
- IBM Spectrum Archive 1.3.1
- Spectrum Protect Policies
Functioning policy and expected behaviour
In general, this automatic process is transparent for the user and you can only notice it when you need to access or modify a file that has been migrated. If the file has been migrated, any access to it will be delayed until its content is retrieved from tape.
- Which files are migrated to tapes and which are not?
Only the files with a size between 1GB and 12TB will be moved (migrated) to tapes from the GPFS disk when no data access and modification have been done for a period of 30 days.
- Working directory (under which copies are made)
/gpfs/archive/hpc
- What happens if I try to modify/delete an already migrated file?
From the user point of view, the deletion will be transparent and have the same behaviour. On the other hand, it is not possible to modify a migrated file; in that case, you will have to wait for the system to retrieve the file and put it back on disk.
- What happens if I’m close to my quota limit?
If there is not enough space to recover a given file from tape, the retrieve will fail and everything will remain in the same state as before, that is, you will continue to see the file on tape (in the “migrated” state).
- How can I check the status of a file?
You can use the hsmFileState script to check if the file is resident on disk or has been migrated to tape..
Examples of use cases
$ hsmFileState file_1MB.dat
resident -rw-rw-r-- 1 user group 1048576 mar 12 13:45 file_1MB.dat
$ hsmFileState file_10GB.dat
migrated -rw-rw-r-- 1 user group 10737418240 feb 12 11:37 file_10GB.dat
Local Hard Drive ↩
Every node has a local solid-state drive that can be used as a local scratch space to store temporary files during executions of one of your jobs. This space is mounted over /scratch/tmp/$JOBID directory and pointed out by $TMPDIR environment variable. The amount of space within the /scratch filesystem is about 1,6 TB. All data stored in theselocal hard drives at the compute nodes will not be available from the login nodes. You should use the directory referred to by $TMPDIR to save your temporary files during job executions. This directory will automatically be cleaned after the job finishes.
Root Filesystem ↩
The root file system, where the operating system is stored, has its own partition.
There is a separate partition of the local hard drive mounted on /tmp that can be used for storing user data as you can read in Local Hard Drive.
NVMe ↩
Every node has two non-volatile memory express drives (3 TB each) that can be used as a local working directory to speed-up the code by reducing notably the access time to disk.
You can use them with the same methodology described for the local hard drive. There are environment variables to refer to the directories mounted on each drive. Use $NVME1DIR and $NVME2DIR to refer to each drive directory.
Quotas ↩
The quotas are the amount of storage available for a user or a groups’ users. You can picture it as a small disk readily available to you. A default value is applied to all users and groups and cannot be outgrown.
You can inspect your quota anytime you want using the following command from inside each filesystem:
% bsc_quota
The command provides a readable output for the quota.
If you need more disk space in this filesystem or in any other of the GPFS filesystems, the responsible for your project has to make a request for the extra space needed, specifying the requested space and the reasons why it is needed. For more information or requests you can Contact Us.
Data management ↩
Transferring files ↩
There are several ways to copy files from/to the Cluster:
- Direct scp, rsync, sftp… to the login nodes
- Using a Data transfer Machine which shares all the GPFS filesystem for transferring large files
- Mounting GPFS in your local machine
Direct copy to the login nodes.
As said before no connections are allowed from inside the cluster to the outside world, so all scp and sftp commands have to be executed from your local machines and never from the cluster. The usage examples are in the next section.
On a Windows system, most of the secure shell clients come with a tool to make secure copies or secure ftp’s. There are several tools that accomplish the requirements, please refer to the Appendices, where you will find the most common ones and examples of use.
Data Transfer Machine
We provide special machines for file transfer (required for large amounts of data). These machines are dedicated to Data Transfer and are accessible through ssh with the same account credentials as the cluster. They are:
- dt01.bsc.es
- dt02.bsc.es
These machines share the GPFS filesystem with all other BSC HPC machines. Besides scp and sftp, they allow some other useful transfer protocols:
- scp
localsystem$ scp localfile username@dt01.bsc.es:
username's password:
localsystem$ scp username@dt01.bsc.es:remotefile localdir
username's password:
- rsync
localsystem$ rsync -avzP localfile_or_localdir username@dt01.bsc.es:
username's password:
localsystem$ rsync -avzP username@dt01.bsc.es:remotefile_or_remotedir localdir
username's password:
- sftp
localsystem$ sftp username@dt01.bsc.es
username's password:
sftp> get remotefile
localsystem$ sftp username@dt01.bsc.es
username's password:
sftp> put localfile
- BBCP
bbcp -V -z <USER>@dt01.bsc.es:<FILE> <DEST>
bbcp -V <ORIG> <USER>@dt01.bsc.es:<DEST>
GRIDFTP (only accessible from dt02.bsc.es)
SSHFTP
globus-url-copy -help
globus-url-copy -tcp-bs 16M -bs 16M -v -vb your_file sshftp://your_user@dt01.bsc.es/~/
Setting up sshfs - Option 1: Linux
Create a directory inside your local machine that will be used as a mount point.
Run the following command below, where the local directory is the directory you created earlier. Note that this command mounts your GPFS home directory by default.
sshfs -o workaround=rename <yourHPCUser>@dt01.bsc.es: <localDirectory>
From now on, you can access that directory. If you access it, you should see your home directory of the GPFS filesystem. Any modifications that you do inside that directory will be replicated to the GPFS filesystem inside the HPC machines.
Inside that directory, you can call “git clone”, “git pull” or “git push” as you please.
Setting up sshfs - Option 2: Windows
In order to set up sshfs in a Windows system, we suggest two options:
sshfs-win
Follow the installation steps from their official repository.
Open File Explorer and right-click over the “This PC” icon in the left panel, then select “Map Network Drive”.
Menu selection - In the new window that pops up, fill the “Folder” field with this route:
\\sshfs\<your-username>@dt01.bsc.es
Example - After clicking “Finish”, it will ask you for your credentials and then you will see your remote folder as a part of your filesystem.
Done! win-sshfs
Install Dokan 1.0.5 (is the version that works best for us)
Install the latest version of win-sshfs. Even though the installer seems to do nothing, if you reboot your computer the direct access to the application will show up.
The configuration fields are:
% Drive name: whatever you want % Host: dt01.bsc.es % Port: 22 % Username: <your-username> % Password: <your-password> % Directory: directory you want to mount % Drive letter: preferred % Mount at login: preferred % Mount folder: only necessary if you want to mount it over a directory, otherwise, empty % Proxy: none % KeepAlive: preferred
Example - After clicking “Mount” you should be able to access to your remote directory as a part of your filesystem.
Done!
Data Transfer on the PRACE Network
PRACE users can use the 10Gbps PRACE Network for moving large data among PRACE sites. To get access to this service it’s required to contact “support@bsc.es” requesting its use, providing the local IP of the machine from where it will be used.
The selected data transfer tool is Globus/GridFTP which is available on dt02.bsc.es
In order to use it, a PRACE user must get access to dt02.bsc.es:
% ssh -l pr1eXXXX dt02.bsc.es
Load the PRACE environment with ‘module’ tool:
% module load prace globus
Create a proxy certificate using ‘grid-proxy-init’:
% grid-proxy-init
Your identity: /DC=es/DC=irisgrid/O=bsc-cns/CN=john.foo
Enter GRID pass phrase for this identity:
Creating proxy ........................................... Done
Your proxy is valid until: Wed Aug 7 00:37:26 2013
pr1eXXXX@dtransfer2:~>
The command ‘globus-url-copy’ is now available for transferring large data.
globus-url-copy [-p <parallelism>] [-tcp-bs <size>] <sourceURL> <destURL>
Where:
-p: specify the number of parallel data connections should be used (recommended value: 4)
-tcp-bs: specify the size (in bytes) of the buffer to be used by the underlying ftp data channels (recommended value: 4MB)
Common formats for sourceURL and destURL are:
- file:// (on a local machine only) (e.g. file:///home/pr1eXX00/pr1eXXXX/myfile)
- gsiftp:// (e.g. gsiftp://supermuc.lrz.de/home/pr1dXXXX/mydir/)
- remember that any url specifying a directory must end with /.
All the available PRACE GridFTP endpoints can be retrieved with the ‘prace_service’ script:
% prace_service -i -f bsc
gftp.prace.bsc.es:2811
More information is available at the PRACE website
Active Archive Management ↩
To move or copy from/to AA you have to use our special commands, available in dt01.bsc.es and dt02.bsc.es or any other machine by loading “transfer” module:
- dtcp, dtmv, dtrsync, dttar
These commands submit a job into a special class performing the selected command. Their syntax is the same than the shell command without ‘dt’ prefix (cp, mv, rsync, tar).
- dtq, dtcancel
dtq
dtq shows all the transfer jobs that belong to you, it works like squeue in SLURM.
dtcancel <job_id>
dtcancel cancels the transfer job with the job id given as parameter, it works like scancel in SLURM.
- dttar: submits a tar command to queues. Example: Taring data from /gpfs/ to /gpfs/archive/hpc
% dttar -cvf /gpfs/archive/hpc/group01/outputs.tar ~/OUTPUTS
- dtcp: submits a cp command to queues. Remember to delete the data in the source filesystem once copied to AA to avoid duplicated data.
# Example: Copying data from /gpfs to /gpfs/archive/hpc
% dtcp -r ~/OUTPUTS /gpfs/archive/hpc/group01/
# Example: Copying data from /gpfs/archive/hpc to /gpfs
% dtcp -r /gpfs/archive/hpc/group01/OUTPUTS ~/
- dtrsync: submits a rsync command to queues. Remember to delete the data in the source filesystem once copied to AA to avoid duplicated data.
# Example: Copying data from /gpfs to /gpfs/archive/hpc
% dtrsync -avP ~/OUTPUTS /gpfs/archive/hpc/group01/
# Example: Copying data from /gpfs/archive/hpc to /gpfs
% dtrsync -avP /gpfs/archive/hpc/group01/OUTPUTS ~/
- dtsgrsync: submits a rsync command to queues switching to the specified group as the first parameter. If you are not added to the requested group, the command will fail. Remember to delete the data in the source filesystem once copied to the other group to avoid duplicated data.
# Example: Copying data from group01 to group02
% dtsgrsync group02 /gpfs/projects/group01/OUTPUTS /gpfs/projects/group02/
- dtmv: submits a mv command to queues.
# Example: Moving data from /gpfs to /gpfs/archive/hpc
% dtmv ~/OUTPUTS /gpfs/archive/hpc/group01/
# Example: Moving data from /gpfs/archive/hpc to /gpfs
% dtmv /gpfs/archive/hpc/group01/OUTPUTS ~/
Additionally, these commands accept the following options:
--blocking: Block any process from reading file at final destination until transfer completed.
--time: Set up new maximum transfer time (Default is 18h).
It is
Repository management (GIT/SVN) ↩
There’s no outgoing internet connection from the cluster, which prevents the use of external repositories directly from our machines. To circumvent that, you can use the “sshfs” command in your local machine, as explained in the previous [Setting up sshfs (Linux)] and [Setting up sshfs (Windows)] sections.
Doing that, you can mount a desired directory from our GPFS filesystem in your local machine. That way, you can operate your GPFS files as if they were stored in your local computer. That includes the use of git, so you can clone, push or pull any desired repositories inside that mount point and the changes will transfer over to GPFS.
Running Jobs ↩
Slurm is the utility used for batch processing support, so all jobs must be run through it. This section provides information for getting started with job execution at the Cluster.
Submitting jobs ↩
The method for submitting jobs is to use the SLURM sbatch directives directly.
A job is the execution unit for SLURM. A job is defined by a text file containing a set of directives describing the job’s requirements, and the commands to execute.
In order to ensure the proper scheduling of jobs, there are execution limitations in the number of nodes and cpus that cna be used at the same time by a group. You may check those limits using command ‘bsc_queues’. If you need to run an execution bigger than the limits already granted, you may contact support@bsc.es.
Important accounting changes
To ensure fair and reliable CPU usage accounting information, we’ve enforced the need to use at least 40 threads for each GPU requested. In your job scripts, make sure that the amount of threads used meet the requirements for your GPU needs. Note that Slurm does refer to each thread as if it was a physical CPU.
The value of “cpu-per-task” x “task-per-node” should amount to those 40 threads. Remember that, by default, the value of “cpu-per-task” is 1.
If you can’t change the number of tasks in your job, you can edit the number of CPUs per task (#SBATCH –cpus-per-task=). In order to not affect your executions, you can choose the desired CPUs per task by setting the environment variable OMP_NUM_THREADS (this variable may not work for every application).
Otherwise, an error message will be displayed pointing out this issue:
sbatch: error: Minimum cpus requested should be (nodes * gpus/node * 40).
Cpus requested: X. Gpus: Y, Required cpus: Z
sbatch: error: Batch job submission failed: CPU count specification invalid
SBATCH commands
These are the basic directives to submit jobs with sbatch:
sbatch <job_script>
submits a “job script” to the queue system (see Job directives).
squeue
shows all the submitted jobs.
scancel <job_id>
remove the job from the queue system, canceling the execution of the processes, if they were still running.
Interactive Sessions ↩
Allocation of an interactive session in the debug partition has to be done through SLURM:
- Interactive session, 64 cores:
salloc -t 00:10:00 -n 1 -c 64 -J debug srun --pty /bin/bash
You may add -c <ncpus> to allocate n CPUs.
Job directives ↩
A job must contain a series of directives to inform the batch system about the characteristics of the job. These directives appear as comments in the job script and have to conform to either the sbatch syntaxes.
sbatch syxtax is of the form:
#SBATCH --directive=value
Additionally, the job script may contain a set of commands to execute. If not, an external script may be provided with the ‘executable’ directive. Here you may find the most common directives for both syntaxes:
# sbatch
#SBATCH --qos=debug
This partition is only intended for small tests.
# sbatch
#SBATCH --time=HH:MM:SS
The limit of wall clock time. This is a mandatory field and you must set it to a value greater than real execution time for your application and smaller than the time limits granted to the user. Notice that your job will be killed after the time has passed.
# sbatch
#SBATCH -D pathname
The working directory of your job (i.e. where the job will run). If not specified, it is the current working directory at the time the job was submitted.
# sbatch
#SBATCH --error=file
The name of the file to collect the standard error output (stderr) of the job.
# sbatch
#SBATCH --output=file
The name of the file to collect the standard output (stdout) of the job.
# sbatch
#SBATCH --ntasks=number
The number of processes to start.
Optionally, you can specify how many threads each process would open with the directive:
# sbatch
#SBATCH --cpus-per-task=number
The number of cpus assigned to the job will be the total_tasks number * cpus_per_task number.
# sbatch
#SBATCH --ntasks-per-node=number
The number of tasks assigned to a node.
# sbatch
#SBATCH --gres=gpu:number
The number of GPU assigned to a node.
#SBATCH --exclusive
To request an exclusive use of a compute node without sharing the resources with other users.
#SBATCH --reservation=reservation_name
The reservation where your jobs will be allocated (assuming that your account has access to that reservation). In some ocasions, node reservations can be granted for executions where only a se t of accounts can run jobs. Useful for courses.
#SBATCH --mail-type=[begin|end|all|none]
#SBATCH --mail-user=<your_email>
#Fictional example (notified at the end of the job execution):
#SBATCH --mail-type=end
#SBATCH --mail-user=dannydevito@bsc.es
Those two directives are presented as a set because they need to be used at the same time. They will enable e-mail notifications that are triggered when a job starts its execution (begin), ends its execution (end) or both (all). The “none” option doesn’t trigger any e-mail, it is the same as not putting the directives. The only requisite is that the e-mail specified is valid and also the same one that you use for the HPC User Portal (what is the HPC User Portal, you ask? Excellent question, check it out here!: https://www.bsc.es/user-support/hpc_portal.php)
#SBATCH --x11=[=<all|batch|first|last>]
If it is set the job will be handled as graphical and Slurm will assign the necessary resources to the job, so you will be able to execute a graphical command and if you do not close the current terminal you will get a graphical window. Sets up X11 forwarding on all, batch host, first or last node(s) of the allocation.
# sbatch
#SBATCH --switches=number@timeout
By default, Slurm schedules a job in order to use the minimum amount of switches. However, a user can request a specific network topology in order to run his job. Slurm will try to schedule the job for timeout minutes. If it is not possible to request number switches (from 1 to 14) after timeout minutes, Slurm will schedule the job by default.
Variable | Meaning |
---|---|
SLURM_JOBID | Specifies the job ID of the executing job |
SLURM_NPROCS | Specifies the total number of processes in the job |
SLURM_NNODES | Is the actual number of nodes assigned to run your job |
SLURM_PROCID | Specifies the MPI rank (or relative process ID) for the current process. The range is from 0-(SLURM_NPROCS–1) |
SLURM_NODEID | Specifies relative node ID of the current job. The range is from 0-(SLURM_NNODES–1) |
SLURM_LOCALID | Specifies the node-local task ID for the process within a job |
Examples
sbatch examples
Example for a sequential job:
#!/bin/bash
#SBATCH --job-name="test_serial"
#SBATCH -D .
#SBATCH --output=serial_%j.out
#SBATCH --error=serial_%j.err
#SBATCH --ntasks=1
#SBATCH --time=00:02:00
./serial_binary> serial.out
The job would be submitted using:
> sbatch ptest.cmd
Example for a parallel job:
#!/bin/bash
#SBATCH --job-name=test_parallel
#SBATCH -D .
#SBATCH --output=mpi_%j.out
#SBATCH --error=mpi_%j.err
#SBATCH --ntasks=20
#SBATCH --cpus-per-task=4
#SBATCH --time=00:02:00
#SBATCH --gres=gpu:2
mpirun ./parallel_binary> parallel.output
Interpreting job status and reason codes ↩
When using squeue, Slurm will report back the status of your launched jobs. If they are still waiting to enter execution, they will be followed by the reason. Slurm uses codes to display this information, so in this section we will be covering the meaning of the most relevant ones.
Job state codes
This list contains the usual state codes for jobs that have been submitted:
- COMPLETED (CD): The job has completed the execution.
- COMPLETING (CG): The job is finishing, but some processes are still active.
- FAILED (F): The job terminated with a non-zero exit code.
- PENDING (PD): The job is waiting for resource allocation. The most common state after running “sbatch”, it will run eventually.
- PREEMPTED (PR): The job was terminated because of preemption by another job.
- RUNNING (R): The job is allocated and running.
- SUSPENDED (S): A running job has been stopped with its cores released to other jobs.
- STOPPED (ST): A running job has been stopped with its cores retained.
Job reason codes
This list contains the most common reason codes of the jobs that have been submitted and are still not in the running state:
- Priority: One or more higher priority jobs is in queue for running. Your job will eventually run.
- Dependency: This job is waiting for a dependent job to complete and will run afterwards.
- Resources: The job is waiting for resources to become available and will eventually run.
- InvalidAccount: The job’s account is invalid. Cancel the job and resubmit with correct account.
- InvaldQoS: The job’s QoS is invalid. Cancel the job and resubmit with correct account.
- QOSGrpCpuLimit: All CPUs assigned to your job’s specified QoS are in use; job will run eventually.
- QOSGrpMaxJobsLimit: Maximum number of jobs for your job’s QoS have been met; job will run eventually.
- QOSGrpNodeLimit: All nodes assigned to your job’s specified QoS are in use; job will run eventually.
- PartitionCpuLimit: All CPUs assigned to your job’s specified partition are in use; job will run eventually.
- PartitionMaxJobsLimit: Maximum number of jobs for your job’s partition have been met; job will run eventually.
- PartitionNodeLimit: All nodes assigned to your job’s specified partition are in use; job will run eventually.
- AssociationCpuLimit: All CPUs assigned to your job’s specified association are in use; job will run eventually.
- AssociationMaxJobsLimit: Maximum number of jobs for your job’s association have been met; job will run eventually.
- AssociationNodeLimit: All nodes assigned to your job’s specified association are in use; job will run eventually.
Software Environment ↩
All software and numerical libraries available at the cluster can be found at /apps/. If you need something that is not there please contact us to get it installed (see Getting Help).
C Compilers ↩
In the cluster you can find these C/C++ compilers :
xlc / xlc++ -> IBM C/C++ Compilers
% xlc --help
% xlc++ --help
gcc / g++ -> GNU Compilers for C/C++
% man gcc
% man g++
pgcc / pgc++ -> Portland Group Compilers for C/C++
% module load pgi
% man gcc
% man g++
All invocations of the C or C++ compilers follow these suffix conventions for input files:
.C, .cc, .cpp, or .cxx -> C++ source file.
.c -> C source file
.i -> preprocessed C source file
.so -> shared object file
.o -> object file for ld command
.s -> assembler source file
By default, the preprocessor is run on both C and C++ source files.
These are the default sizes of the standard C/C++ datatypes on the machine
Type | Length (bytes) |
---|---|
bool (c++ only) | 1 |
char | 1 |
wchar_t | 4 |
short | 2 |
int | 4 |
long | 8 |
float | 4 |
double | 8 |
long double | 16 |
GCC
The GCC provided by the system is version 6.4.0. For better support of new and old hardware features we have different versions that can be loaded via the provided modules. For example in Power you can find GCC 9.2.0
module load gcc/9.2.0
Distributed Memory Parallelism
To compile MPI programs it is recommended to use the following handy wrappers: mpicc, mpicxx for C and C++ source code. You need to choose the Parallel environment first: module load openmpi / module load ibm_mpi. These wrappers will include all the necessary libraries to build MPI applications without having to specify all the details by hand.
% mpicc a.c -o a.exe
% mpicxx a.C -o a.exe
Shared Memory Parallelism
OpenMP directives are supported by the GNU/PGI C and C++ compilers. To use it, the flag -fopenmp/-mp must be added to the compile line.
% gcc -fopenmp -o exename filename.c
% g++ -fopenmp -o exename filename.C
You can also mix MPI + OPENMP code using -fopenmp/-mp with the mpi wrappers mentioned above.
FORTRAN Compilers ↩
In the cluster you can find these compilers :
xlf -> IBM Fortran Compiler
% xlf -qhelp
gfortran -> GNU Compilers for FORTRAN
% man gfortran
pgfortran -> Portland Group Compilers for Fortran
% module load pgi
% man pgfortran
By default, the compilers expect all FORTRAN source files to have the extension “.f”, and all FORTRAN source files that require preprocessing to have the extension “.F”. The same applies to FORTRAN 90 source files with extensions “.f90” and “.F90”.
Distributed Memory Parallelism
In order to use MPI, again you can use the wrappers mpif77 or mpif90 depending on the source code type. You can always man mpif77 to see a detailed list of options to configure the wrappers, ie: change the default compiler.
% mpif77 a.f -o a.exe
Shared Memory Parallelism
OpenMP directives are supported by the GNU/PGI Fortran compiler when the option -fopenmp/-mp is set:
% gfortran -fopenmp
% pgfortran -mp
Modules Environment ↩
The Environment Modules package (http://modules.sourceforge.net/) provides a dynamic modification of a user’s environment via modulefiles. Each modulefile contains the information needed to configure the shell for an application or a compilation. Modules can be loaded and unloaded dynamically, in a clean fashion. All popular shells are supported, including bash, ksh, zsh, sh, csh, tcsh, as well as some scripting languages such as perl.
Installed software packages are divided into five categories:
- Environment: modulefiles dedicated to prepare the environment, for example, get all necessary variables to use openmpi to compile or run programs
- Tools: useful tools which can be used at any time (php, perl, …)
- Applications: High Performance Computers programs (GROMACS, …)
- Libraries: Those are tipycally loaded at a compilation time, they load into the environment the correct compiler and linker flags (FFTW, LAPACK, …)
- Compilers: Compiler suites available for the system (intel, gcc, …)
Modules tool usage
Modules can be invoked in two ways: by name alone or by name and version. Invoking them by name implies loading the default module version. This is usually the most recent version that has been tested to be stable (recommended) or the only version available.
% module load pgi
Invoking by version loads the version specified of the application. As of this writing, the previous command and the following one load the same module.
% module load pgi/18.1
The most important commands for modules are these:
- module list shows all the loaded modules
- module avail shows all the modules the user is able to load
- module purge removes all the loaded modules
- module load <modulename> loads the necessary environment variables for the selected modulefile (PATH, MANPATH, LD_LIBRARY_PATH…)
- module unload <modulename> removes all environment changes made by module load command
- module switch <oldmodule> <newmodule> unloads the first module (oldmodule) and loads the second module (newmodule)
You can run “module help” any time to check the command’s usage and options or check the module(1) manpage for further information.
BSC Commands ↩
The Support team at BSC has provided some commands useful for user’s awareness and ease of use in our HPC machines. A short summary of these commands follows:
- bsc_queues: Show the queues the user has access to and their time/resources limits.
- bsc_quota: Show a comprehensible quota usage summary for all accessible filesystems.
- bsc_load: Displays job load information across all related computing nodes.
All available commands have a dedicated manpage (not all commands are available for all machines). You can check more information about these commands checking their respective manpage:
% man <bsc_command>
For example:
% man bsc_quota
Getting help ↩
BSC provides users with excellent consulting assistance. User support consultants are available during normal business hours, Monday to Friday, 09 a.m. to 18 p.m. (CEST time).
User questions and support are handled at: support@bsc.es
If you need assistance, please supply us with the nature of the problem, the date and time that the problem occurred, and the location of any other relevant information, such as output files. Please contact BSC if you have any questions or comments regarding policies or procedures.
Our address is:
Barcelona Supercomputing Center – Centro Nacional de Supercomputación
C/ Jordi Girona, 31, Edificio Capilla 08034 Barcelona
Frequently Asked Questions (FAQ) ↩
You can check the answers to most common questions at BSC’s Support Knowledge Center. There you will find online and updated versions of our documentation, including this guide, and a listing with deeper answers to the most common questions we receive as well as advanced specific questions unfit for a general-purpose user guide.
Appendices ↩
SSH ↩
SSH is a program that enables secure logins over an insecure network. It encrypts all the data passing both ways, so that if it is intercepted it cannot be read. It also replaces the old an insecure tools like telnet, rlogin, rcp, ftp,etc. SSH is a client-server software. Both machines must have ssh installed for it to work.
We have already installed a ssh server in our machines. You must have installed an ssh client in your local machine. SSH is available without charge for almost all versions of UNIX (including Linux and MacOS X). For UNIX and derivatives, we recommend using the OpenSSH client, downloadable from http://www.openssh.org, and for Windows users we recommend using Putty, a free SSH client that can be downloaded from http://www.putty.org. Otherwise, any client compatible with SSH version 2 can be used. If you want to try a simpler client with multi-tab capabilities, we also recommend using Solar-PuTTY (https://www.solarwinds.com/free-tools/solar-putty).
This section describes installing, configuring and using PuTTy on Windows machines, as it is the most known Windows SSH client. No matter your client, you will need to specify the following information:
- Select SSH as default protocol
- Select port 22
- Specify the remote machine and username
For example with putty client:
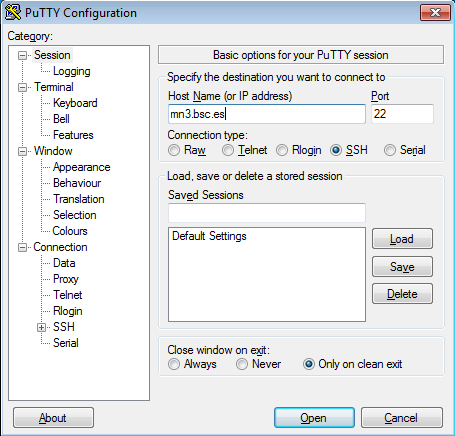
This is the first window that you will see at putty startup. Once finished, press the Open button. If it is your first connection to the machine, your will get a Warning telling you that the host key from the server is unknown, and will ask you if you are agree to cache the new host key, press Yes.
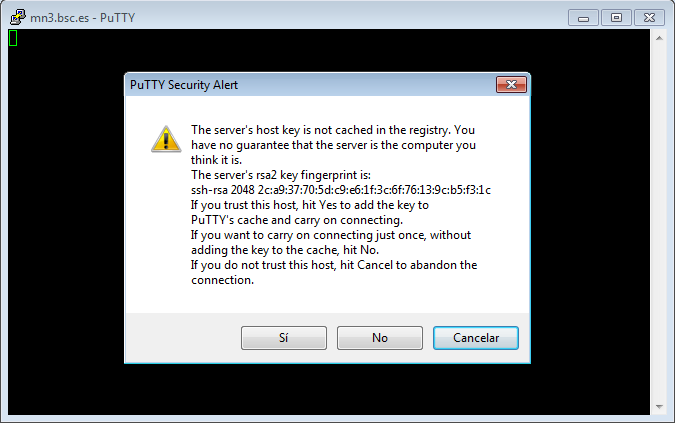
IMPORTANT: If you see this warning another time and you haven’t modified or reinstalled the ssh client, please do not log in, and contact us as soon as possible (see Getting Help).
Finally, a new window will appear asking for your login and password:
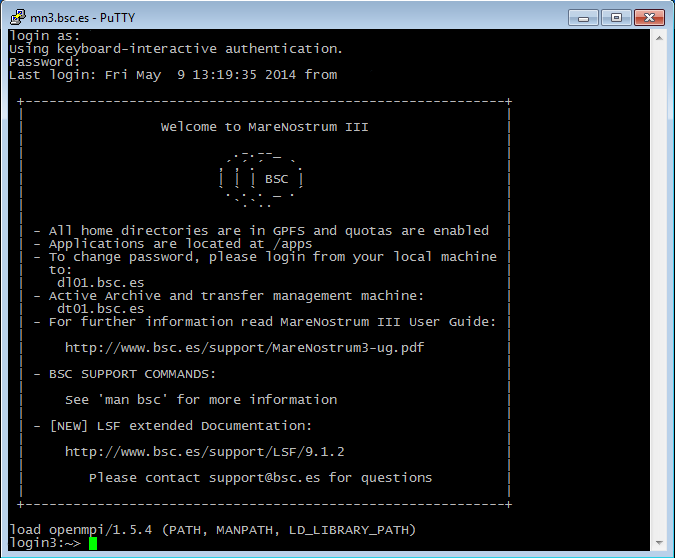
Generating SSH keys with PuTTY
First of all, open PuTTY Key Generator. You should select Type RSA and 2048 or 4096 bits, then hit the “Generate” button.
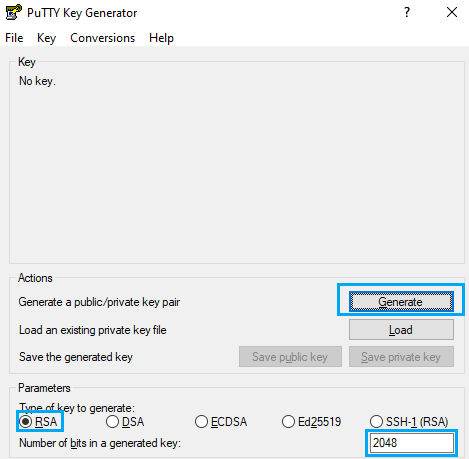
After that, you will have to move the mouse pointer inside the blue rectangle, as in picture:

You will find and output similar to the following picture when completed

This is your public key, you can copy the text in the upper text box to the notepad and save the file. On the other hand, click on “Save private key” as in the previous picture, then export this file to your desired path.
You can close PuTTY Key Generator and open PuTTY by this time,
To use your recently saved private key go to Connection -> SSH -> Auth, click on Browse… and select the file.

Transferring files on Windows ↩
To transfer files to or from the cluster you need a secure FTP (SFTP) o secure copy (SCP) client. There are several different clients, but as previously mentioned, we recommend using the Putty clients for transferring files: psftp and pscp. You can find them at the same web page as PuTTY (http://www.putty.org), you just have to go to the download page for PuTTY and you will see them in the “alternative binary files” section of the page. They will most likely be included in the general PuTTY installer too.
Some other possible tools for users requiring graphical file transfers could be:
- WinSCP: Freeware SCP and SFTP client for Windows (http://www.winscp.net)
- Solar-PuTTY: Free alternative to PuTTY that also has graphical interfaces for SCP/SFTP. (https://www.solarwinds.com/free-tools/solar-putty)
Using PSFTP
You will need a command window to execute psftp (press start button, click run and type cmd). The program first asks for the machine name (mn1.bsc.es), and then for the username and password. Once you are connected, it’s like a Unix command line.
With command help you will obtain a list of all possible commands. But the most useful are:
- get file_name : To transfer from the cluster to your local machine.
- put file_name : To transfer a file from your local machine to the cluster.
- cd directory : To change remote working directory.
- dir : To list contents of a remote directory.
- lcd directory : To change local working directory.
- !dir : To list contents of a local directory.
You will be able to copy files from your local machine to the cluster, and from the cluster to your local machine. The syntax is the same that cp command except that for remote files you need to specify the remote machine:
Copy a file from the cluster:
> pscp.exe username@mn1.bsc.es:remote_file local_file
Copy a file to the cluster:
> pscp.exe local_file username@mn1.bsc.es:remote_file
Using X11 ↩
In order to start remote X applications you need and X-Server running in your local machine. Here are two of the most common X-servers for Windows:
- Cygwin/X: http://x.cygwin.com
- X-Win32 : http://www.starnet.com
The only Open Source X-server listed here is Cygwin/X, you need to pay for the other.
Once the X-Server is running run putty with X11 forwarding enabled:

On the other hand, XQuartz is the most common application for this purpose in macOS. You can download it from its website:
For older versions of macOS or XQuartz you may need to add these commands to your .zshrc file and open a new terminal:
export DISPLAY=:0
/opt/X11/bin/xhost +
This will allow you to use the local terminal as well as xterm to launch graphical applications remotely.
If you installed another version of XQuartz in the past, you may need to launch the following commands to get a clean installation:
$ launchctl unload /Library/LaunchAgents/org.macosforge.xquartz.startx.plist
$ sudo launchctl unload /Library/LaunchDaemons/org.macosforge.xquartz.privileged_startx.plist
$ sudo rm -rf /opt/X11* /Library/Launch*/org.macosforge.xquartz.* /Applications/Utilities/XQuartz.app /etc/*paths.d/*XQuartz
$ sudo pkgutil --forget org.macosforge.xquartz.pkg
I tried running a X11 graphical application and got a GLX error, what can I do?
If you are running on a macOS/Linux system and, when you try to use some kind of graphical interface through remote SSH X11 remote forwarding, you get an error similar to this:
X Error of failed request: BadValue (integer parameter out of range for operation)
Major opcode of failed request: 154 (GLX)
Minor opcode of failed request: 3 (X_GLXCreateContext)
Value in failed request: 0x0
Serial number of failed request: 61
Current serial number in output stream: 62
Try to do this fix:
macOS:
- Open a command shell, type, and execute:
$ defaults find xquartz | grep domain
You should get something like ‘org.macosforge.xquartz.X11’ or ‘org.xquartz.x11’, use this text in the following command (we will use org.xquartz.x11 for this example):
$ defaults write org.xquartz.x11 enable_iglx -bool true
- Reboot your computer.
Linux:
- Edit (as root) your Xorg config file and add this:
Section "ServerFlags"
Option "AllowIndirectGLX" "on"
Option "IndirectGLX" "on"
EndSection
- Reboot your computer.
This solves the error most of the time. The error is related to the fact that some OS versions have disabled indirect GLX by default, or disabled it at some point during an OS update.
Requesting and installing a .X509 user certificate ↩
If you are a BSC employee (and you also have a PRACE account), you may be interested in obtaining and configuring a x.509 Grid certificate. If that is the case, you should follow this guide. First, you should obtain a certificate following the details of this guide (you must be logged in the BSC intranet):
Once you have finished requesting the certificate, you must download it in a “.p12” format. This procedure may be different depending on which browser you are using. For example, if you are using Mozilla Firefox, you should be able to do it following these steps:
- Go to “Preferences”.
- Navigate to the “Privacy & Security” tab.
- Scroll down until you reach the “Certificates” section. Then, click on “View Certificates…”
- You should be able to select the certificate you generated earlier. Click on “Backup…”.
- Save the certificate as “usercert.p12”. Give it a password of your choice.
Once you have obtained the copy of your certificate, you must set up your environment in your HPC account. To acomplish that, follow these steps:
- Connect to dt02.bsc.es using your PRACE account.
- Go to the GPFS home directory of your HPC account and create a directory named “.globus”.
- Upload the .p12 certificate you created earlier inside that directory.
- Once you are logged in, insert the following commands (insert the password you chose when needed):
module load prace globus
cd ~/.globus
openssl pkcs12 -nocerts -in usercert.p12 -out userkey.pem
chmod 0400 userkey.pem
openssl pkcs12 -clcerts -nokeys -in usercert.p12 -out usercert.pem
chmod 0444 usercert.pem
Once you have finished all the steps, your personal certificate should be fully installed.